1Data
Improving the Lives of Humans and Animals
What can we learn from animals to help humans and vice versa? Working together across species and organizations to improve human and animal health will help enhance research and, ultimately, improve the lives of all species.
1Data is a unique partnership by Kansas State University and the University of Missouri-Kansas City. It leverages the strengths of our region and partners in the health industry to develop a structured data platform to share human and animal health research data.
Initiatives on the 1Data plaform
1DrugAssist is an intelligent medicine recommender system (IMRS) for patients suffering from breast cancer, diabetes, etc. It suggests the most effective therapeutic options for treating a patient's metastatic breast cancer or diabetes by using machine-learning based on computational models that account for various risk factors and an individual's demographic information.
gFARAD serves as the primary source for scientifically-based recommendations regarding safe withdrawal intervals of drugs and chemicals in food-producing animals. As such, it is a key resource for protection of America's food supply, including meat, milk and eggs, against accidental contamination of animal-derived foods with violative residues of drugs, pesticides or other agents that could compromise food safety.
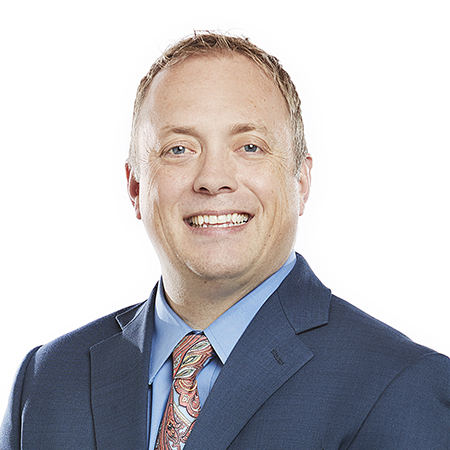
"The 1Data platform collaboration is a shining example of combining the region's unique strengths in human and animal health into an initiative that can transform and improve the quality of life."
— Dennis Ridenour, BioNexus KC CEO
Contact Us